Accountable AI and d/acc
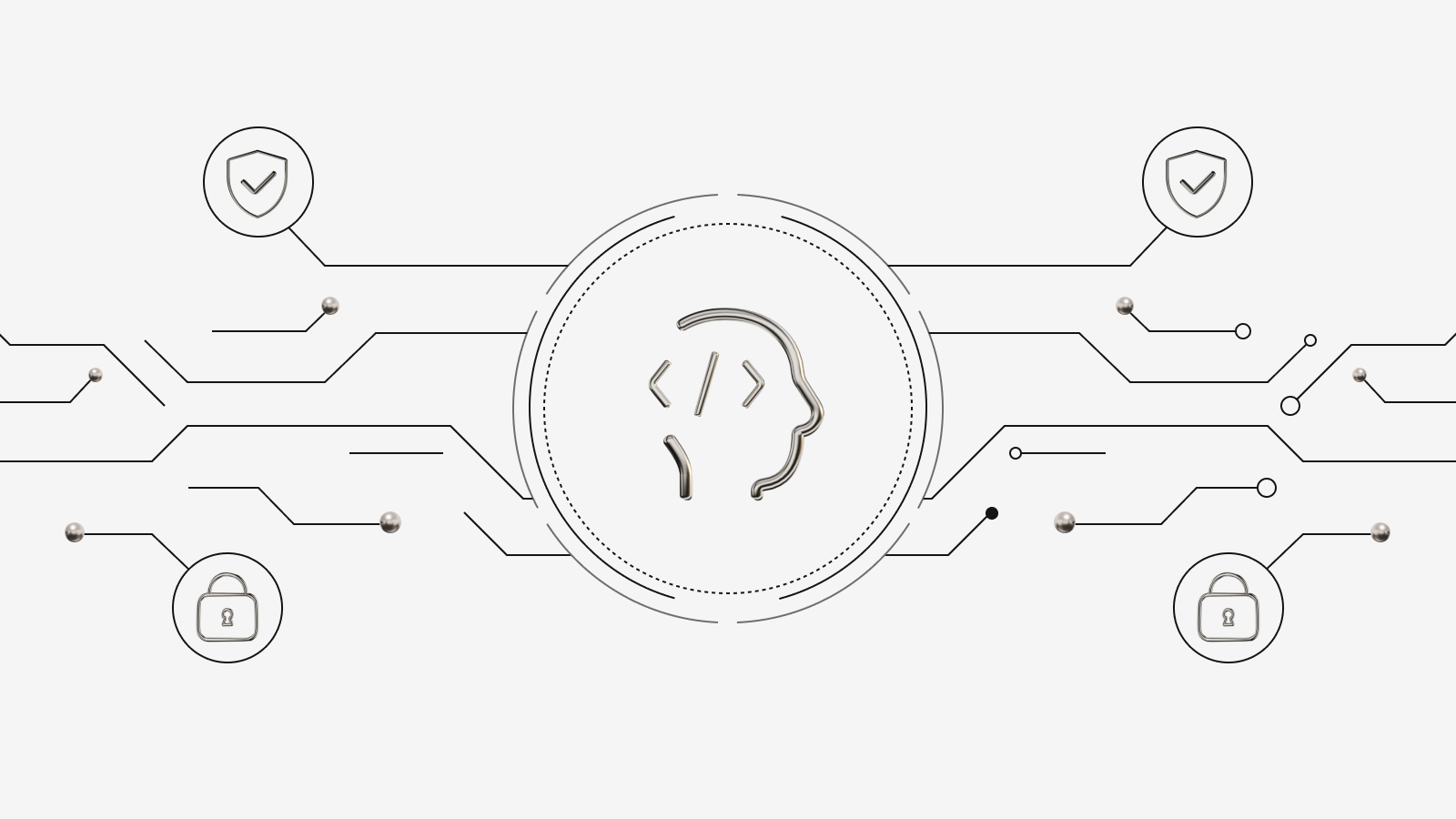
The concept of d/acc (decentralized defensive acceleration) has gained significant traction recently, highlighting a critical but often overlooked aspect of AI development: accountability through cryptographic technologies. While we possess the technical foundations for creating accountable AI systems, we face a familiar challenge that perfectly exemplifies why we need d/acc: the gap between having solutions and achieving widespread adoption.
The Current State: A Tale of Two Realities
Today's reality presents a stark contrast. On one side, we have breakthrough developments in cryptographic technologies - zkML, MPC, and other innovations regularly emerge from research labs, promising to make AI systems more accountable and verifiable. On the other side, these solutions remain largely theoretical, failing to achieve the scale and speed necessary for meaningful real-world impact.
The core issue isn't technological limitations - it's a complex interplay of market dynamics and public perception. While AGI development attracts massive investment due to its clear path to monetization through products and services, defensive measures and accountability infrastructure struggle to attract funding despite their critical importance. Most users and organizations view AI accountability as important but difficult to monetize, leading to a severe underinvestment in these protective technologies. This creates a troubling cycle:
- Limited investment in accountability infrastructure
- Delayed technological progress
- Slower development of efficient solutions
- Even less attractive adoption prospects
The Science Fiction Paradox
Perhaps the greatest irony lies in how science fiction has shaped public perception. While countless books and movies have explored scenarios of rogue AI, they've inadvertently undermined serious discussions about AI accountability. These narratives, instead of raising genuine awareness, have pushed legitimate concerns into the realm of fiction.
Even more ironic is how these stories often centered on the eternal debate between good and evil AI. Now, as we stand on the brink of presumably beneficial AGI, the lack of serious discussion about safeguarding against malicious AI uses seems particularly shortsighted. The very scenarios that filled our entertainment have somehow failed to translate into practical concern for real-world AI systems.
Learning from Post-Quantum Cryptography: A Parallel Journey
Our current situation mirrors the Post-Quantum Cryptography (PQC) landscape from a decade ago. Twenty years ago, PQC development was making steady progress, but faced widespread indifference. Few understood or cared about its implications for web infrastructure, despite the existence of viable technical solutions.
Today's accountable AI technologies face remarkably similar challenges. As the team behind the first zkML paper introducing zero-knowledge decision trees and current holder of the world record for zkML inference speed, we've witnessed firsthand how computational overhead becomes a barrier to adoption. Just as with early PQC candidates, we have working solutions that many consider too expensive in terms of performance, even though our solution has improved over 10,000 times over the past several years.
The PQC story offers a crucial lesson: what ultimately drove change wasn't primarily technical innovation. The core algorithms remained largely unchanged - a stark example is the Falcon signature scheme, which still requires exactly 666 bytes today, the same as it did 8 years ago. Instead, NIST's standardization efforts created the institutional momentum necessary for acceptance. Today, organizations readily accept PQC solutions with similar performance characteristics that were once considered dealbreakers. This acceptance is now reaching blockchain platforms, with Ethereum considering PQC implementation and other major projects following suit - a clear signal that the technology has crossed the threshold from theoretical concern to practical necessity.
However, we envision a different trajectory for Accountable AI. Unlike PQC, we're still making significant technical breakthroughs each year. With continued momentum, we believe these solutions can achieve adoption much faster than PQC's timeline.
Why We Can't Wait: The d/acc Imperative
We cannot afford to repeat the decade-long adoption curve of PQC. The stakes with AI are simply too high. This is precisely where the d/acc framework proves invaluable. By emphasizing the acceleration of defensive technologies while maintaining decentralization, d/acc provides a blueprint for parallel advancement of both technical capabilities and adoption.
A prime example where accountable AI technologies like zkML could make immediate impact is in bio defense and healthcare - an area that the d/acc movement has identified as critically important for humanity's future. As noted in recent d/acc discussions, we face increasing risks from both natural and engineered pathogens, driven by factors like urbanization, global travel, and advancing biotechnology. In this context, hospitals could run AI diagnostics with clear accountability trails - if the AI system makes a mistake, the hospital can prove it followed proper procedures and protocols, protecting medical professionals from undue liability while still maintaining patient data privacy. This same framework enables vaccine development to leverage worldwide data while maintaining strict privacy controls.
This is just one example from a broad range of potential applications where accountable AI can provide immediate, tangible benefits. However, we can't realize these benefits without proper investment and adoption.
Our team continues to push the boundaries of zkML, but technical innovation alone isn't enough. We need a coordinated effort that:
- Accelerates research and development to reduce performance overhead
- Initiates standardization efforts early, learning from PQC's success
- Builds practical awareness disconnected from science fiction scenarios
- Creates concrete incentive structures for adopting accountable AI systems
- Maintains decentralization to prevent the concentration of control
Notably, the path to adoption differs between Web2 and Web3 ecosystems. For Web2, standardization and legislation play a crucial role in driving adoption. In contrast, Web3 adoption hinges more on creating the right incentive structures - a natural fit for blockchain-based systems where economic alignment is built into the architecture.
The Path Forward
The d/acc framework isn't just a philosophical stance - it's a practical approach to ensuring AI development proceeds safely without sacrificing progress. We need to:
- Develop benchmarks and standards for AI accountability
- Create open-source implementations of key technologies
- Build developer tools that make integration straightforward
- Establish industry consortiums to drive adoption
- Engage with policymakers to create supportive regulatory frameworks
This isn't about preventing theoretical worst-case scenarios - it's about building AI systems we can trust and verify today. The time for action is now, before we find ourselves facing the consequences of unaccountable AI systems at scale.
As we push forward with AI development, let's ensure we're not just making systems more powerful, but also more accountable. The technical foundations exist - our challenge is to transform them into widely-adopted solutions before it's too late.